Over the past months, Large Language Models (LLMs) have increasingly received a lot of attention both from the general public and research organizations, as well as organizations worldwide, irrespective of their size.
In essence, LLMs are deep learning models that operate on the scope of natural language processing and natural language generation. These models are able to learn the complexity of human language, and therefore their potential is immense, with applications being currently developed for text classification and sentiment analysis, language translation or speech recognition, customer support (chatbots and question-answering), and text-to-image generation or image captioning.
Across all sectors – from finance, telecom, healthcare, and transportation – organizations have been trying to leverage the application of LLMs to their business models. However, behind the vast potential of these models, there is also a risk of delivering biased and inaccurate results, with potentially nefarious consequences for business outcomes – loss of revenue, lost opportunities, and even brand and reputational damage.
The reason is that, traditionally, the tendency has been to train these models with large amounts of data, based on the assumption that “the more data, the better”, and therefore neglecting the quality of data to some extent. However, within the Data-Centric AI paradigm, we are now aware that “more is not necessarily better”, and that data quality plays a vital role in the success of AI applications.
Data Quality is key for LLMs
Data Quality may refer to a wide range of components regarding the data at hand, and while some data quality issues are standard across most applications and domains, others depend on the specific nature or type of the data, or even the downstream task that is using that data.
Overall, real-world datasets are subjected to several issues which highly impact the training process of LLMs. Some examples are missing data, which compromises the learning stages of the models, or imbalance data, which biases the learning task to more represented concepts, neglecting or producing erroneous outputs for others.
To be able to detect, highlight, and handle them appropriately, having tools that provide a comprehensive analysis of the data – namely data profiling – is essential for success.
Data Profiling is the process of automatically determining the main characteristics of the data, from basic statistics to detailed visualizations – to help data professionals identify and mitigate issues associated with their datasets.
YData has been shaping the way that organizations and professionals move towards more data-centric approaches, focusing on improving their data prior to putting too much time and effort into models that will not have the expected return on investment.
With the ydata-profiling package, YData has helped several data professionals learn about the principles of data-centric AI and educate the stakeholders and decision-makers within their companies on the beneficial impact of an automated and comprehensive data profiling process.
For larger and more complex use cases, or to attend to specific requirements and constraints of organizations, YData offers a more complete and specialized solution for data profiling within YData Fabric. Fabric’s Data Catalog supports organizations with an accurate and comprehensive management and understanding of their data, improving the collaboration, communication, and productivity of the data science teams.
Among the key features of the Data Catalog, the ability to provide a detailed overview of the dataset’s metadata and deliver an extensive, automated, and standardized data profiling are key for developing LLMs, guaranteeing that the applications return accurate and reliable outputs that align with the business objectives.
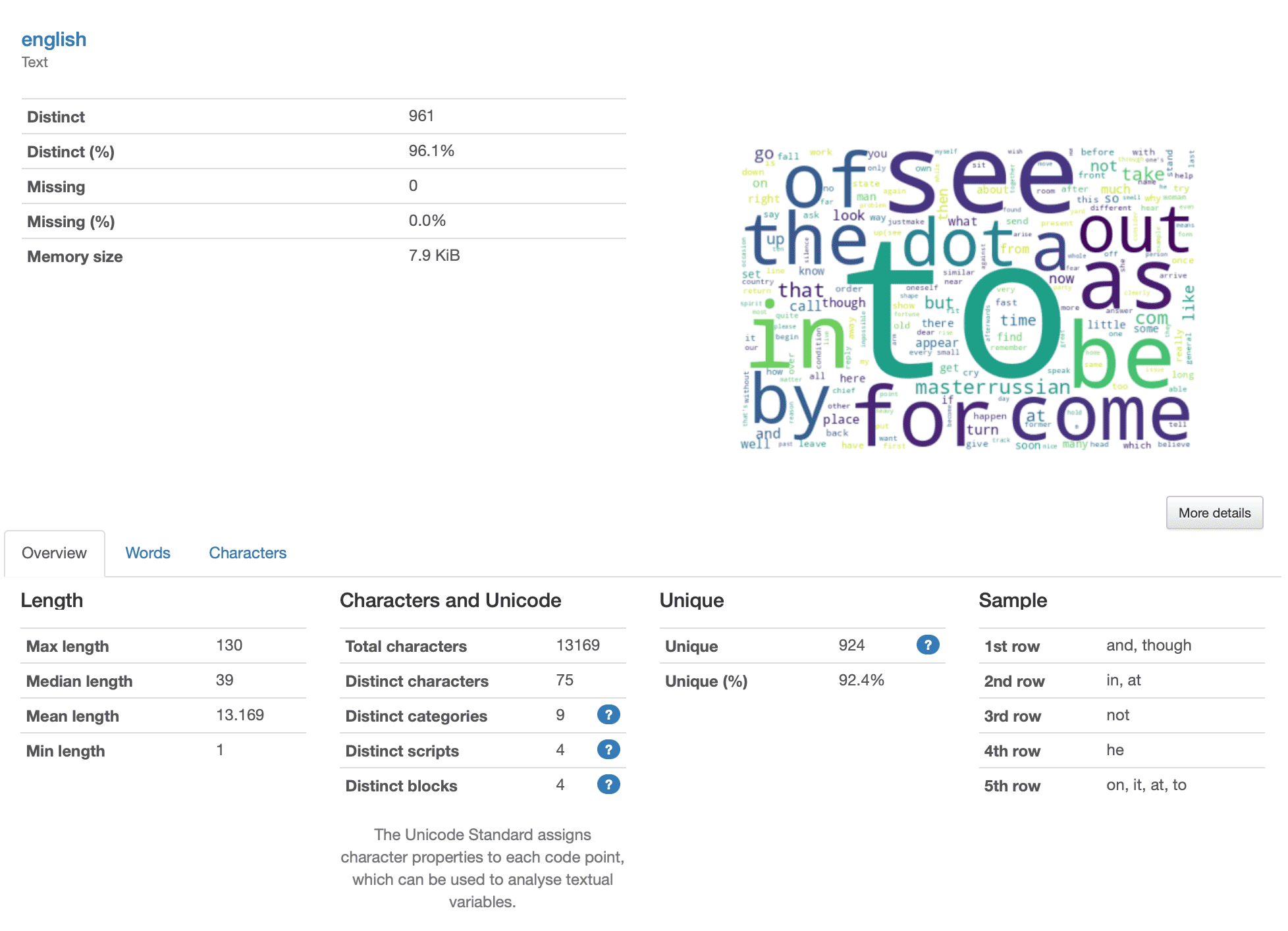
Conclusion
Data Quality is at the heart of all AI/ML applications, and LLMs are no exception. These models are taking all sectors of technology by storm, changing several facets of our daily lives, and will continue to evolve rapidly.
However, to unlock their full potential, guaranteeing high-quality and biased data is critical, where data profiling stands as the most essential task to align business requirements with the available data and expected outcomes.
Start creating better data for your LLMs with YData Fabric Catalog. You can learn more about its benefits with our introductory video and sign up for the community version to get the full experience of building your models with high-quality data.
Find us at the Data-Centric AI Community and join the revolution of high-quality data for faster AI.
Cover Photo by Patrick Tomasso on Unsplash