The importance of data quality & profiling for the success of Machine Learning
In today's world, businesses around the globe are generating a vast amount of data. To be able to adopt a data-driven initiative, organizations must manage data efficiently and effectively. Nevertheless, it can be challenging to do it effectively.
For the success of Analytics solutions such as dashboards or data engineering flows, a structured system must be in place so information is easy to locate, explore and manage. The exact requirements apply to Machine Learning (ML).
Data Catalogs can make a difference!
This article will explore the importance of a Data Catalog, its benefits, and its role in developing successful AI. Understand and discover what makes YData Fabric Catalog unique and necessary in any data science workload with a deep dive into its features and capabilities.
The importance of Data Catalogs
Imagine that in a project, you fail to track the location of critical data - you have no idea where you can get it, what information it holds, nor with whom that dataset is shared. The number of CSV and ad-hoc "storage" of data can be daunting and prejudicial to drawing fast and reliable conclusions for the business - missing data, lack of consistency and coherence about the data that is being used, duplicated efforts, and, the worst part, compliance risks.
A Data Catalog solves this problem by allowing organizations to locate and understand their data faster and more efficiently. Through a centralized data repository, a data catalog improves productivity and understandably of the information and enables data literacy.
What is a data catalog?
Accordingly to Gartner, “A data catalog creates and maintains an inventory of data assets through the discovery, description, and organization of distributed datasets. The data catalog provides context to enable data stewards, data/business analysts, data engineers, data scientists and other lines of business (LOB) data consumers to find and understand relevant datasets for the purpose of extracting business value.”
In simpler terms, a data catalog is comparable to a library or inventory of an organization's datasets. Data Catalogs provide a place where all the data is neatly organized, setting the historical details and lineage while keeping the data ready to be used.
Why data science initiatives need data catalogs?
Data catalogs enable data-driven decision-making and promote data governance while improving data management efficiency.
For any Data Science or ML project, there is always the need to ingest, search, access, and understand the available datasets. Furthermore, there is a significant component associated with the fit and validity of the data that needs to be assessed depending on the context of an application.
The particular data quality needs for Machine Learning led to a new breed of data catalogs that enable:
Fast and unified data discoverability
ML models heavily rely on data, and a data catalog can help data scientists and ML practitioners faster access and ease of discoverability of the datasets that they need in the modeling tasks. Data catalogs provide a centralized and unified repository for the datasets required in a project, enabling alignment in what concerns ML projects' requirements.
Efficiency in data exploration
Performant ML models require a deep understanding of the data being used. A data catalog designed to support ML development provides detailed metadata about the datasets, including descriptions, tags for ease of identification, schema information including variable and data types, and summarized and actionable data quality metrics.
Furthermore, a visual and interactive exploration experience is key for data scientists to comprehend the data's structure, content, and limitations, enabling them to make informed decisions during the model development process.
Improved data quality
Data quality is paramount in ML. The choice of a proper data catalog solution can deliver the data quality metrics and indicators to support data scientists in the fitness assessment of datasets for ML tasks.
Data quality metrics return potential issues and inconsistencies within the data, such as missing values, outliers, or unexpected values, which may require pre-processing or cleaning steps before feeding the data into ML algorithms.
Guided Feature Engineering and data preparation
In any ML model development, feature engineering is a crucial step involving the selection, transformation, and creation of input features. A data catalog is the solution that eases the process of feature engineering more interactively while providing insights into the available features in different datasets, allowing data scientists to identify which are the relevant features and how they can be used as inputs to their ML models.
Combining feature engineering with a proper data catalog saves time and effort for development teams, as it keeps all the information about what features were created and the improvements they bring without having to start from scratch.
Ease of data provenance identification
Understanding the provenance, storage, and how data is manipulated within the organization is crucial for developing data-driven solutions.
Data catalogs provide the needed information to ensure compliance and auditing, mapping how the data usage while ensuring is handled while ensuring that it is being handled ethically and responsibly.
Better data reproducibility and collaboration
ML projects require collaboration among data scientists, researchers, engineers, and even business stakeholders. A data catalog enables reproducibility as all the data sources explored and used to develop ML models are uniquely documented, delivering a unified perspective.
Data profiling significantly speeds up innovation, problem-solving, and decision-making processes, as consistency and simplified knowledge-sharing are guaranteed.
By implementing and adopting a data catalog, the efficiency and effectiveness of ML projects are enhanced with the full support of data discovery, profiling, feature engineering, and data quality assessment. Data Catalogs designed for Data science initiatives improve the overall governance and management and enable better decision-making while fostering collaboration and reproducibility, which is so important for models' development, deployment, and maintenance.
What is unique about Fabric's Catalog?
Fabric: The data catalog to accelerate data discovery and understanding!
The choice and implementation of a Data Catalog for ML initiatives can be a game changer for businesses of all sizes. It is crucial for a faster and better understanding of the available data and how valuable it can be, leading to better decision-making and improved outcomes.
Fabric's Catalog is the catalyst that accelerates and standardizes the first step of any ML project and includes the following features:
- Easy data ingestion:
The Catalog from Fabric provides different connectors that make connecting and setting up data consumption from various storage options easy. It includes RDBMs like MySQL, PostgreSQL, Azure SQL, and object storage such as Google Cloud Storage or AWS S3.
Fabric's Catalog is a combination of Connectors and Data sources leading to improved data management by enabling collaboration between different teams and profiles. This can also streamline governance processes, making them more efficient.
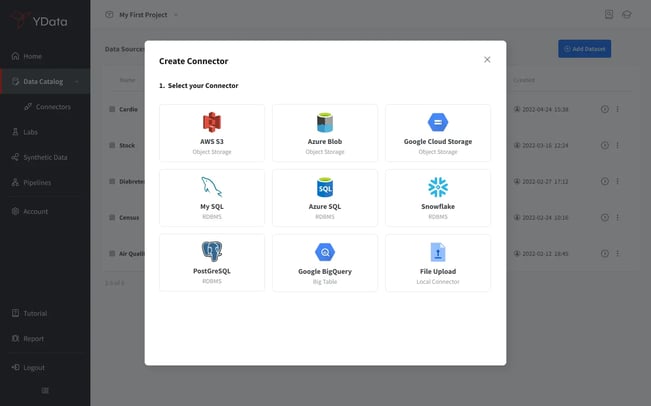
YData Fabric list of connectors experience
- A curated and discoverable list of datasets per project:
A comprehensive and easy-to-access list of available datasets for a data science project enables data teams to gain a unified view of all the available data for development. Fabric's Catalog accelerates access to the right data, allowing more time for proper data analysis. It also helps to foster collaboration among the users involved in the project development.
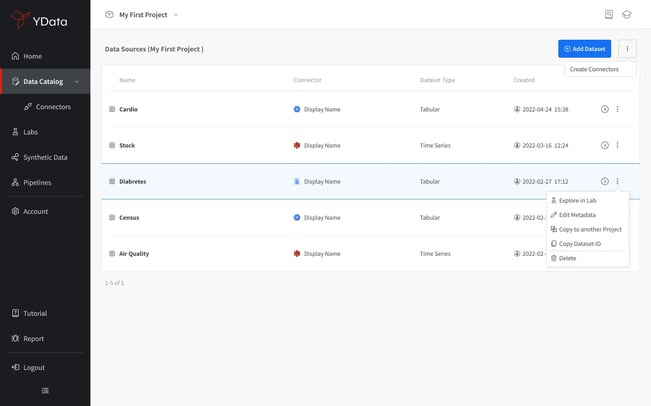
List of available datasets for a project in Fabric's Catalog
- An overview and detailed metadata dataset:
Fabric's Catalog includes comprehensive and complete metadata of your data. It provides information that goes from the provenance of your data to detailed statistics in an interactive experience. Users can instantly see the source of the data, the business context through descriptions and tags.
The data discovery and analysis process is speed-up with an opinionated warning section, letting the users understand the most significant data quality challenges.
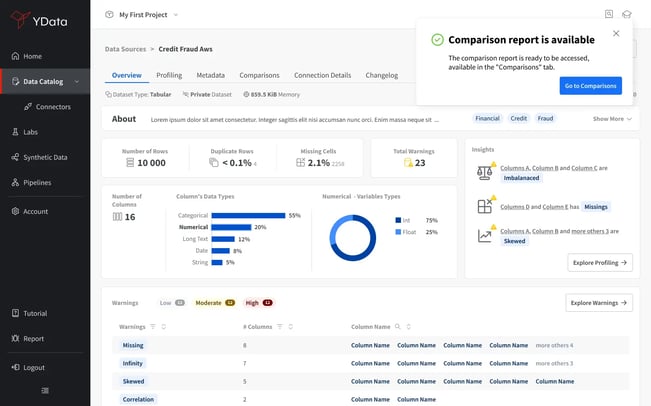
Datasource overview in Fabric's Catalog
- Extensive data profiling for thorough and standardized data quality assessments:
But the greatest value of Fabric's Data Catalog is the detailed and complete analysis of every ingested dataset. A complete exploratory data analysis combined with a data quality check can accelerate data science development up to 5 times. Furthermore, it makes a project more predictable regarding the steps and stages required for successful completion.
Explore from correlations all the way to your missing data behavior!
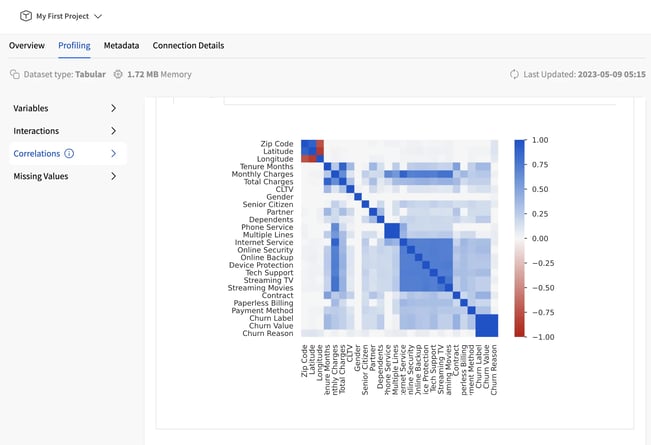
Deep-dive into data source characteristics - missing values, correlations, etc
Conclusion
A data catalog sits at the heart of data governance, quality, and management. It enables businesses to locate, access, and analyze company data faster and more efficiently. The benefits of data catalogs extend far beyond finding data. It's time to unlock the true potential of your business's data with a reliable data catalog solution.
Explore the benefits of YData Fabric Catalog in our short explanatory video. Learn how our powerful features can optimize your workflows and improve your results.
But why stop there? Sign-up for our community version and accelerate your development process.