Cover Photo by Philipp Katzenberger on Unsplash
In the last decade, we’ve increasingly focused on model-centric Artificial Intelligence, building ever more flexible machine learning models. However, a new paradigm shift – Data-Centric AI – is currently revolutionizing the industry, as organizations quickly realize that “more is not always better”. Collecting and storing tons of data without being able to share it or use it freely, or having data that simply does not meet the basic requirements of quality or regulatory compliance has virtually no value.
Data-Centric AI – a new paradigm that focuses on continuously and systematically improving data quality – is reshaping the way businesses operate, enabling them to rapidly accelerate AI development in an efficient and responsible manner. In this new paradigm, high-quality data (rather than models) is seen as the most valuable asset for businesses in their pursuit of success.
In this article, we’ll go over the main best practices for organizations to effectively leverage their data assets.
Best Practices to leverage your Data as an Asset
1. Improving Data Management
Data should be readily available and easily accessible to those who need it. Having a structured system where data teams can locate, explore, and manage the organization’s data is fundamental for the development of effective data engineering and analytic flows.
With Fabric’s Data Catalog, data teams can get on the same page regarding the data being used within a specific project or initiative and which information each dataset holds through a centralized repository. This promotes a unified and transparent data ecosystem and empowers teams to extract insights and make informed decisions swiftly and effectively.

Fabric's Data Catalog
2. Ensuring Data Quality
Artificial Intelligence solutions are only as good as the data they’re trained with. Real-world data is often plagued with several quality issues, such as imbalanced data, missing data, and outliers, among others. Poor-quality data can lead to incorrect predictions and biased decision-making, with severe consequences for business outcomes. To truly leverage data as an asset, you need to thoroughly know your data characteristics and behavior.
Fabric provides a comprehensive and scalable profiling of your organization’s data, allowing data professionals to explore data quality metrics and indicators in order to profile, clean, and enrich the existing assets for machine learning tasks.
3. Enabling Accurate and Unbiased Data Preparation
Biased data can lead to discriminatory AI outcomes, impacting your business's reputation and potentially leading to legal consequences. Biased data can arise from issues during data collection and selection, but it can also arise intrinsically from the nature of the domain itself. Being able to identify potential bias issues and enabling a thorough data preparation is key to guarantee that your data is free from discriminatory elements.
Fabric is the first Data-Centric AI platform that focuses on continuous and systematic data improvement. Combining its extensive profiling capabilities with smart synthetic data, you can regain control of your data quality and train your AI models on diverse and representative datasets to enhance fairness and accuracy. Check out our latest Conditional Sampling approach to de-bias and augment training data.
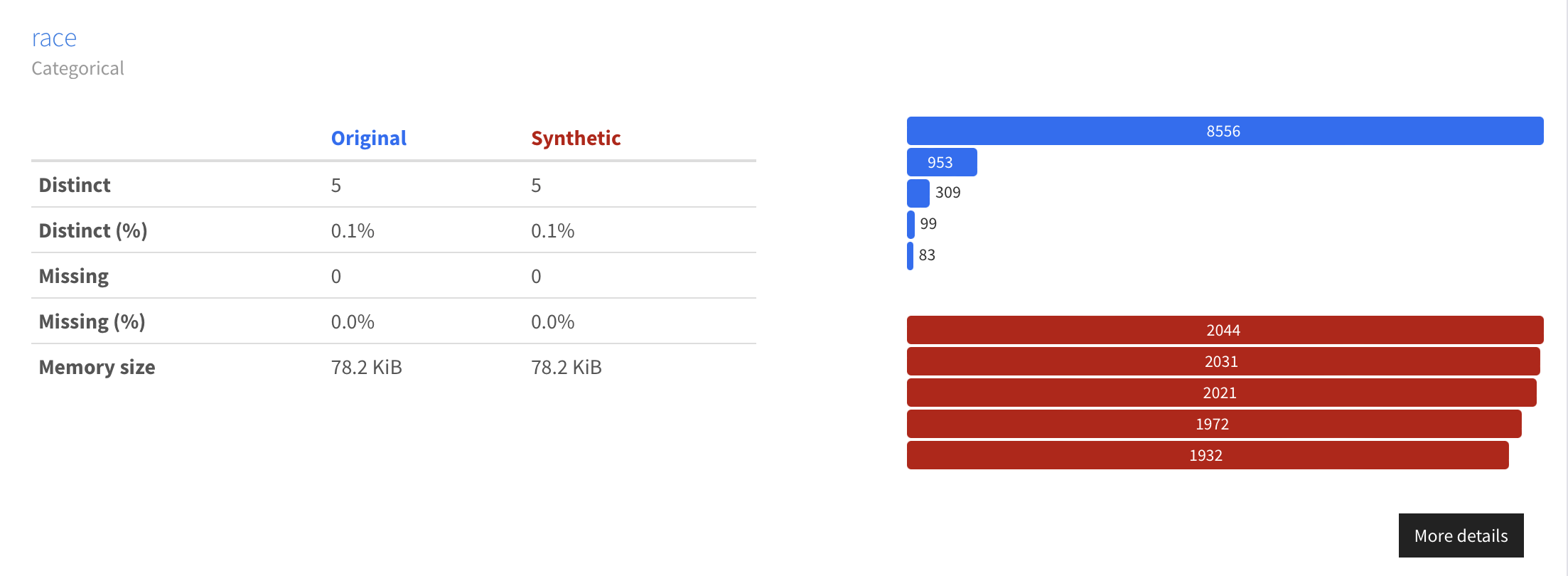
Conditional Sampling is ideal for de-bias, augmentation, and balancing
4. Fostering Data Sharing, Data Privacy, and Security
Data sharing is a fundamental necessity to ensure that organizations can take the most out of their data and put themselves in front of their peers regarding business value metrics. Although initiatives such as GDPR and CCPA have helped formulate better frameworks for protecting private data, they have also increased the fear of sharing data, even within organizations, and created a bottleneck in AI development, slowing down data teams.
Some strategies to mitigate this issue include anonymization and masking, although they cannot solve the problem entirely. Instead, Fabric leverages smart synthetic data that complies with privacy regulations. The synthesizers are available for tabular and time-series data and for relational databases, enabling you to map your existing data into a synthetic version and unlock your sharing and development initiatives.
Conclusion
In the era of Data-Centric AI, businesses that can effectively leverage their data as an asset will gain a significant competitive advantage.
Maintaining a thorough data catalog, striving for high-quality data, enabling accurate and unbiased data preparation, allowing customization, and prioritizing data privacy are the cornerstones of a successful data strategy.
Only by systematically embracing these strategies can your business harness the transformative power of Data-Centric AI, delivering innovative solutions, enhancing customer experiences, and staying ahead in a rapidly evolving landscape.
Take the leap toward the Data-Centric AI paradigm with Fabric and sign up for the community version to make data your most valuable asset.
Feel free to contact us for trial access to the full platform and have a chat about how to use your data to your advantage. You can also find additional support and more resources at the Data-Centric AI Community.