The creation of new business models and the need to find the right competitive edge is the drive for the growing adoption of AI initiatives. Digital transformation is a heavy legacy transformation and not a 6 months project, nevertheless, organizations understand the relevance of data and its management. Companies need to have access to data and be able to make predictive and prescriptive insights.
Although we are currently witnessing exponential growth both in data volume and interest in a data-based economy, access to data is still a key obstacle to the development of Machine Learning (ML) and Analytics. The truth is that although initiatives such as GDPR, CCPA, and others similar, have helped to institute important regulations for the use of sensitive and private data, they have also increased the fear of sharing data, even within different departments of the same organization.
There are traditional anonymization techniques, but even those have shown not to be enough to ensure privacy under the standards and definitions of regulations.
First, the combination of several other variables and external information can highly increase the risk of re-identification.
Secondly, masking and hashing can lead to the loss of relevant information for developing new business revenue strategies.
In this regard, synthetic data has proven to foster a culture of data-sharing within organizations, overcoming the limitations of traditional privacy methods and maximizing data value.
The importance of data-sharing for businesses
As stated by Gartner in a recent article, data sharing is a business necessity to accelerate digital transformation, with organizations that promote data sharing expected to outperform their peers in terms of business value metrics.
Although this is exciting, in reality, very few data-sharing programs have been created successfully throughout the last few years. The culture of “not sharing” and keeping data private and protected through data silos is inhibiting even internal departments of developing successful data-driven initiatives.
On the other hand, those that have embraced a data-sharing strategy, have reported astonishing improvements. In the end, a once-considered useless dataset can gain extra value when combined with other sets of information. In the realm of e-commerce, companies use external data sources such as weather and traffic patterns to optimize their delivery routes. Banking institutions also rely on data, working with merchants to identify and prevent fraudulent transactions more accurately. Additionally, insurance companies use telemetry and GPS data to personalize insurance offerings and optimize their business operations.
Yet, the benefits of sharing data go far beyond new business revenue models and increase of data value for monetization strategies - it can also foster better and faster innovation, as well as increase customer retention and acquisition.
The easier it gets to share data, the more meaningful is its value, leading to the development of new solutions in a more timely manner. This is crucial for traditional businesses to remain competitive against their digital native competitors.
Synthetic data as a privacy-enhancer
The key to fostering a data-sharing culture
Synthetic data is artificially generated data that keeps the original data properties, while ensuring its business value and privacy compliance. But what makes synthetic data the perfect privacy-preserving technology to enable data sharing?
Since synthetic data records are not real, they do not qualify as personal data. This means that regulations and laws that are meant to protect personal data, such as GDPR, do not apply. Furthermore, because synthetic data records have no one-to-one match in real data, identity disclosure is considered to be much harder, and in some cases, even impossible.
Additionally, synthetic data generated through data-driven mechanisms is not only statistically similar to the real data, but also holds the same utility.
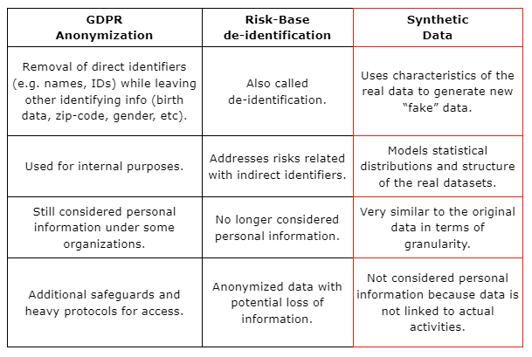
Table 1 - Comparison between Anonymization and Synthetic Data
In conclusion, synthetic data is the most effective solution for enhancing privacy in data sharing, as shown on the comparison table above. It allows organizations to share information while still protecting sensitive and personal information. However, it is important to note that the use of synthetic data alone does not guarantee compliance with privacy regulations and organizations must still follow best practices and guidelines set out by regulatory bodies.
YData Fabric synthesizers
Fabric synthesizers support the synthesis of tabular and time-series data, as well as the ability to replicate full databases.
In order to guarantee the best results for the use case, testing and comparing the newly generated synthetic data and having a report with the results is highly pivotal to provide information on the quality and privacy of synthetic data. YData's generated synthetic data is measured against the following pillars: privacy, fidelity, and utility. The computed scores answer the following core questions for the data-sharing process:
1. Privacy, is my synthetic data leaking any real data information?
2. Fidelity, how does the synthetic data preserve the original data properties?
3. Utility, how well does the synthetic data behave when put into use in a downstream ML application?
Considering privacy as a measure of maximum importance for data sharing, YData’s report metrics measure identity disclosure and attribution risk to mitigate risk and maximize the benefits of synthetic data for data sharing while protecting the privacy of individuals. These refer to the risk that the synthetic data may reveal or allow the identification of individuals, even if the original data has been modified or anonymized. All scores are calculated for every trained synthesizer and delivered as a PDF report for easy sharing within organizations. More details can be found here.
Combined with the Constrain Engine, which applies business rules to the generated data, and the Anonymization engine, which replaces data labeled as PII with new mock generated data, YData Fabric’s synthesis flow is perfect for ensuring a proper data-sharing strategy.