The answer is pretty obvious, right? Let’s take a deeper look at the P&C business.
Like any other business nowadays, artificial intelligence also became a vital aspect of modern Insurance. Insurance companies seat on a gold mine of data, which have potential to generate actionable insights for a variety of use cases.
Today I bring you some of the key use cases for the Property & Casualty segment in the Insurance sector:
Fraud Detection
According to the Insurance Information Institute, fraud comprises about 10 percent of property-casualty insurance losses and loss adjustment expenses each year - the number is around $30 billion yearly. Predictive analysis and deep learning-based applications are being integrated into the Core Claims systems to automate the fraud detection and investigation workflows. You can imagine the impact this can have in a company if these new AI-based techniques increase the accuracy of detecting fraud in some percentage, even if it’s just one digit.
Intelligent Underwriting
Insurance is nothing but transfer of risk in return of premium. In today’s world, insurance premiums are mostly static. But with the advancement of big data and IoT, insurers are able to gather massive volume of both batch and real time data to determine the risk exposure of the insured using predictive modelling. For example, the insurance renewal premium for automobile claims can be increased or decreased based on the driving pattern of the insured. Maybe you should reconsider your driving style, right?
Faster Claims Processing
Claims is another area where artificial intelligence helps insurers to maintain their brand reputation and help their customers in time of needs. For example, you have bought automobile policy from the organization X and unfortunately ran in to a minor accident and broke the wind screen. Now, you can go to the insurer’s website / app / virtual assistant to file a claim and they will ask you to take photos of your broken wind screen. After you upload the photos, in seconds it will automatically determine the claim amount and can also create an appointment with third party vendors for repairs. Deep learning is being used to predict the repair amount based on automatically assessing the damage from the images you uploaded. Cool, right?
Smart Assistance with conversational AI
Days are gone when you need to go to a human agent, understand the terms and conditions and fill up lengthy forms to buy an insurance. Digitally empowered customers are expecting similar level of user experience as chatting in social media while buying an insurance product. Conversational AI systems like social media chatbots, virtual smart assistants are introduced to offer quick quotes, handling customer queries, feedback, etc. On one hand, it helps insurers to reduce operational costs and, on other hand, it offers consumers flexibility to buy an insurance while browsing social media.
Targeted Marketing and Recommendations
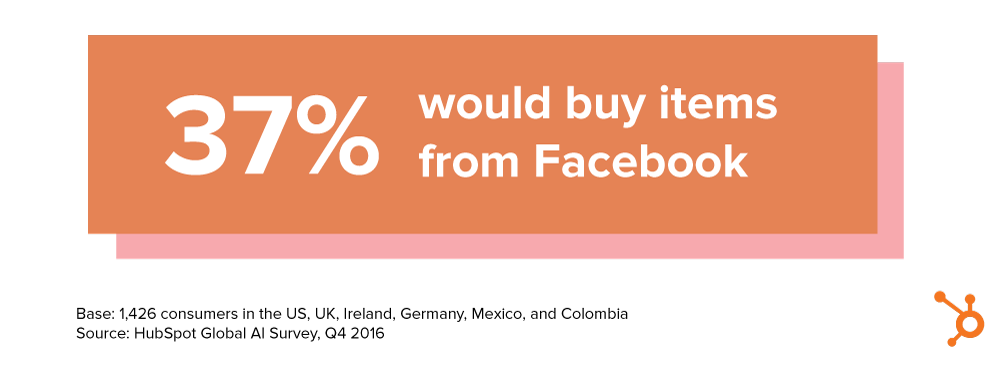
Predictive analysis helps insurers to identify the customer segments which have more likelihood to buy or renew an insurance policy. Based on this analysis, insurers can keep their marketing targeted and reduce costs. Another important use case is to recommend personalized insurance products to these targeted segment of consumers, for instance, leveraging unsupervised machine learning. Personalized insurance offerings will help consumers to choose the product tailored to their lifestyle and will give the carriers opportunity to target new segment of consumers with faster delivery. According to a study by MarketsandMarkets, the recommendation engine market is expected to grow from $588.9 Million in 2016 to $4414.8 Million by 2022, at a Compound Annual Growth Rate (CAGR) of 40.7%. Impressive numbers, but companies are still struggling with the implementation of many of these solutions.
Implementation Challenges
Although Insurance always have been a data driven industry, there are still many challenges to implement some machine learning use cases. These cases may vary from company to company, as the data governance in place can differ, but in general these are the main challenges:
-
Many insurers still run on legacy claims ecosystems, which makes it difficult to integrate with analytics workflows. However, in recent times many P&C carriers are movings towards cloud native ecosystems, such as Duck Creek OnDemand, Guidewire, and others.
-
Insurers deals with sensitive data and, in most cases, acquiring and transforming the data to a useful format with adhering the compliance makes the process complex and lengthy. To reduce the gap, insurance companies are leveraging Privacy Enhancing Technologies, data quality tools such as labelling, or the novel advances in synthetic data. Synthetic data, although not the standard yet, can play a major role not only in enhancing the quality of existing data, but also ensuring compliance with privacy regulations.
-
Lack of data-driven culture and proper data governance often restricts carriers to harvest the positive returns from investing in AI.
Conclusion
Although Insurance companies have decision support systems in place, there’s still a lot to do to make them data-driven. New technologies such as deep learning, conversational AI or synthetic data can play a big role in turning a challenger into market leader. In this article, we briefly discussed some data science use cases in the property & casualty insurance landscape and the challenges to implement them. Every new technology or process comes with newer set of challenges alongside lot of opportunities. With the data revolution and rapidly emerging platforms, artificial intelligence has the potential to transform the P&C market at scale.
Gonçalo Martins Ribeiro is CEO at YData.
Improved and synthetic data for AI
YData offers a data-centric development platform for Data Scientists to work to high-quality and synthetic data.