One good thing about the current pandemic (probably the only good thing) is that everyone stopped spending time commuting and got to spend that time on something else. We’re glad that some of those people were kind enough to spend that time with us, over videoconference.
When building a startup, it’s crucial to be constantly talking with future users or subject matter experts. In our case, data scientists. If you’re a data scientist, you know that 100 interviews are not enough data to take insights from. However, we managed to interview people from all over the world, from Singapore to the USA, from Brazil to Russia. We don’t want to be biased in our analysis, so we embraced this challenge and took it where our time allowed us.
100 conversations wouldn’t be enough if the answers were totally disparate. It happened that ALL of them were quite homogeneous — there are HUGE problems in current data science processes. Most companies claim they leverage machine learning for something. Executives give talks and are quoted by top research firms on how they increase revenue or reduced costs using AI. But, when talking with the ones actually doing the work, the story is quite different!
It seems there’s a huge GAP from the top levels of the companies (executives) and the lower levels (technical people). We see execs claiming they have tons of data to work with and they’re putting it to use, while data scientists claim they don’t have usable data. Where do we stand? Who is telling the truth?
Next time an executive asks you the size of the database/data lake/data warehouse, don’t give them the number before asking why. Or just tell them that size doesn’t matter (pun intended!)
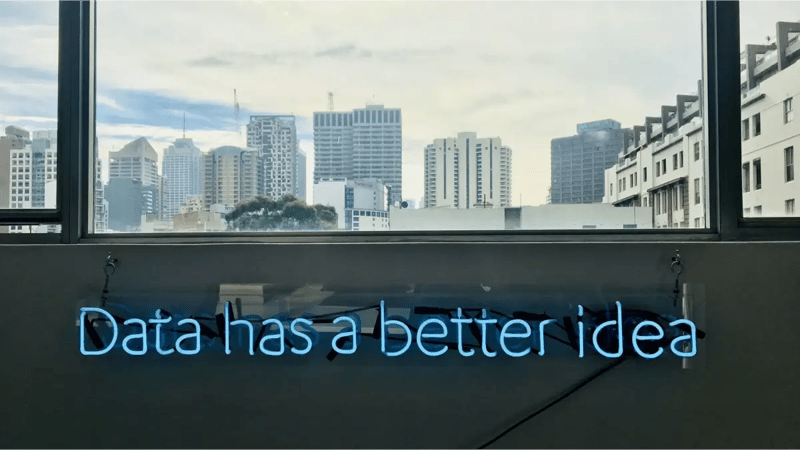
Photo by Franki Chamaki on Unsplash
We went to seek the truth. We spent hours talking and taking notes and today we’re glad to show you a glimpse of those insights. Bear in mind that these were not interviews to prove an hypothesis, rather we spent quality time with peers and discussed the state of data science and machine learning. Here are some quotes:
“The hardest part of being a data scientist in a growing company is to be able to correctly manage and monitor the different data sources. Data is always changing across different areas, when changes are not correctly tracked there might be impacts on the models developed with the data."
Data Scientist from USA
“GDPR is our main problem while working with data, for 2 reasons: Due to GDPR, all our infrastructure is on-prem. This makes the process of productizing machine learning more tedious and challenging. Second, we are not able to access all the data that we need. Data is siloed and the access to it is not granted.”
Data Scientist from Netherlands
“Access to data is a true headache. Hard access to data is not only a problem for data science work, but is also a path to not solve bias issues: if you can only access the data of your reality, your data will be biased.”
AI Researcher from Canada
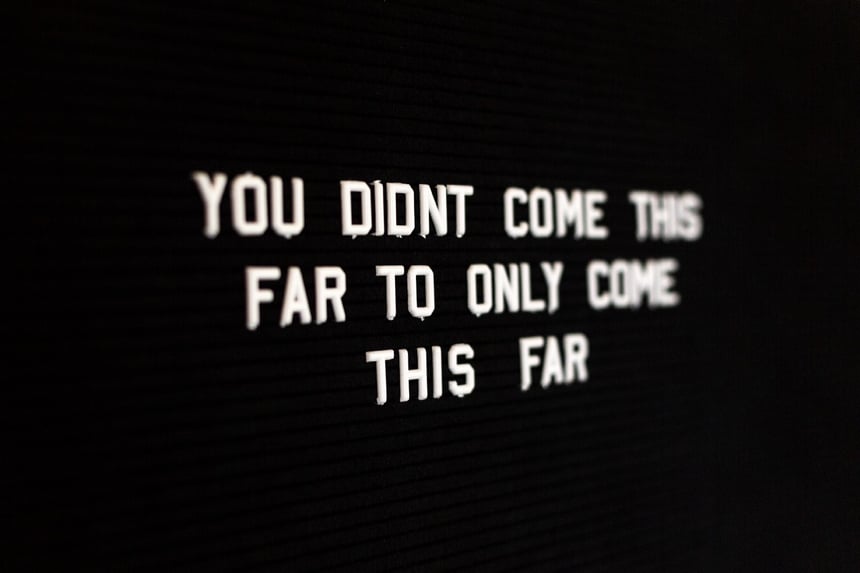
Photo by Drew Beamer on Unsplash
“When I was a researcher in academia, I thought that access to data was hard. Now that I’m in the private sector, I realize it is much worse here.”
Data Scientist from Brazil
“After the SingHealth (Data breach of Singapore health patients), most organizations have secured and locked their data and no one can touch it.”
Senior Data Scientist from Singapore
“Different insights can be extracted whenever there’s no standard exploratory data analysis. This is a real issue, and this leads to wrong insights extraction.”
Founder at a Privacy Preserving Startup from Canada.
As you could read, there’s a clear communication gap in most companies. Data does NOT always exist in high quantity nor quality! It is not even easily accessible!
Most companies are hiring entire data science teams without even knowing if there’s enough data to work with or ensuring data governance processes to at least let them get access to data in the first place.
The biggest insight from all of these conversations was that companies are focused on putting models into production but failing at getting the right data to build those models. Who never tried to start building a house from the roof, right? If data is the prime matter, we have to give it more attention before starting feeding it into models.
(…) companies are focused on putting models into production but failing at getting the right data to build those models.
I’ll leave you with another quote, this time authored:
“The problem isn’t the algorithm, but the dirty data fed into it.”
Anna Bethke, Head of AI for Social Good at Intel
If you are up to a virtual coffee to share your views on the topic, feel free to reach out to me!
Gonçalo Martins Ribeiro is CEO at YData
Improved and synthetic data for AI
YData offers a data experimentation platform with synthetic data generation